AI-powered reporting and annotation for radiology
Accelerate the development and deployment of medical imaging AI with DICOM-native data annotation tools. Supercharge your reporting workflows with AI and unlock extra efficiency and productivity.
Leapfrog into the future of medical AI workflows
Supercharge clinical reporting workflows with LLMs to unlock extra efficiency and productivity. Automatic template selection, key findings dictation mapping, impression generation, proofreading, and more. Streamline administrative tasks with automated billing code generation. Improve patient communication and education with patient-friendly audio messages.
Simple HL7/DICOM integration with EHR/HIS/RIS
Works on desktop, laptop, tablet, or mobile
Multi-device sync
AI-driven or traditional reporting modes
Multilingual support
Contextual AI chat
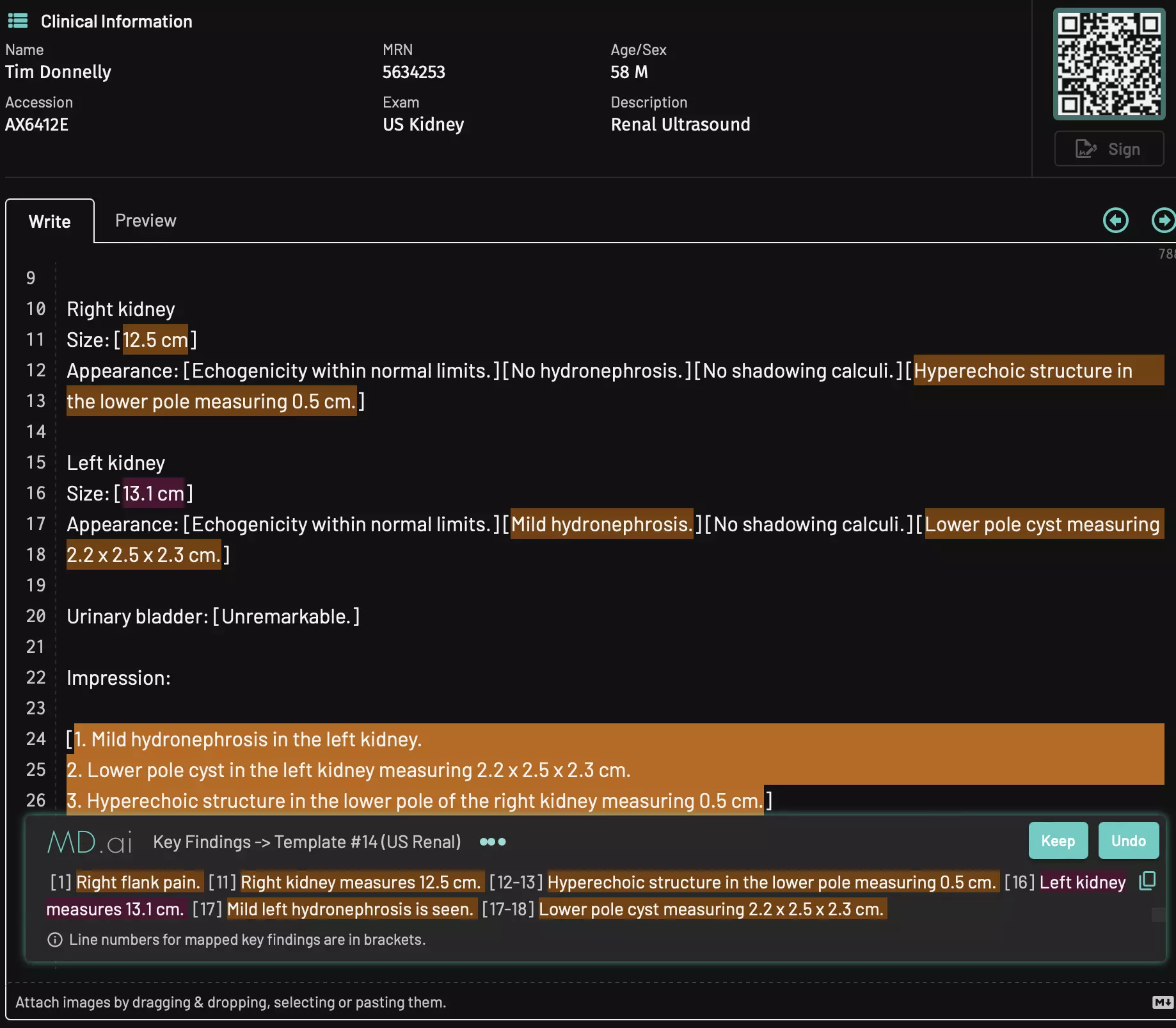
Build high-quality datasets and models
Our platform has enabled thousands of doctors and their collaborators to create large high-quality labeled datasets, deploy and validate their models, and build AI-driven clinical workflows.
Native DICOM support
FDA 510(k)-cleared viewer
Seamless scaling
AI-assisted annotation
PHI detection and De-ID
Developer APIs
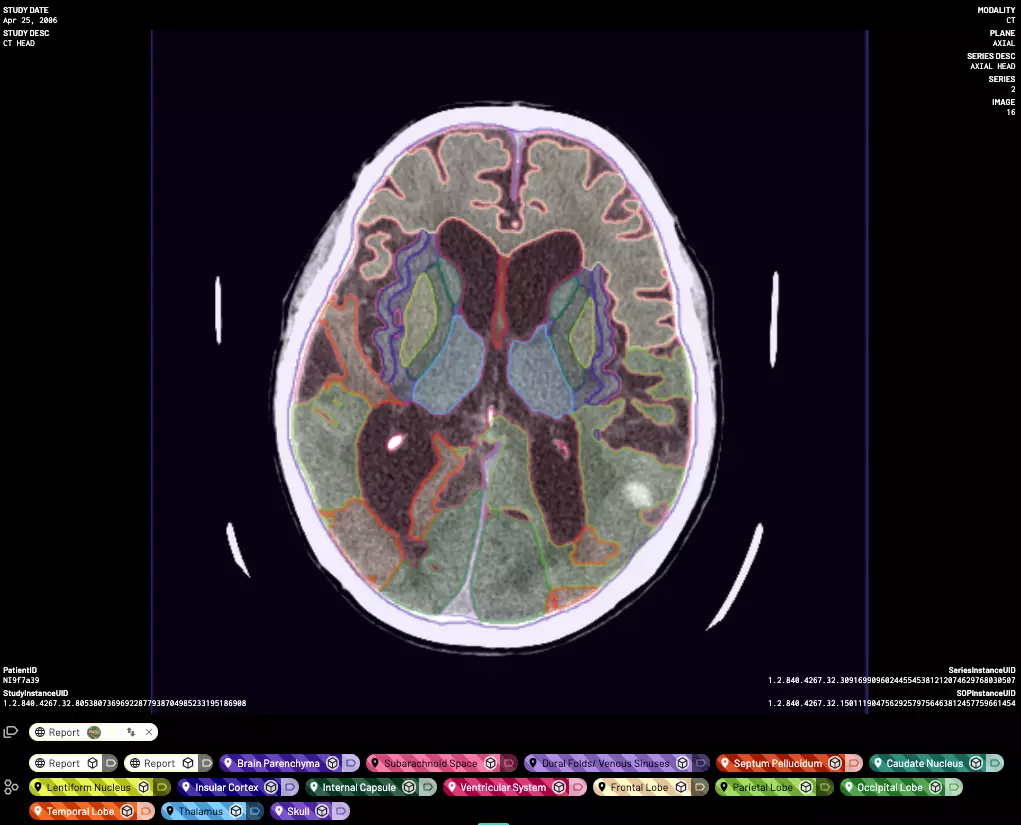
Contact Us
Question for MD.ai? Send a message: email us at hello@md.ai, or fill out the form below.